Did you know that by 2025, the market for computer vision in smart devices and deep learning, including Microsoft Edge AI and Starkey Livio Edge AI, is projected to reach a staggering $1.12 billion? Data scientists will play a crucial role in this growing industry. Edge AI, also known as edge intelligence, is revolutionizing the world of artificial intelligence by bringing its capabilities directly to edge devices. This includes leveraging Microsoft Edge AI, cutting-edge AI, and forward-edge AI to power the inference engine for efficient inferencing. This edge AI solution utilizes deep learning and computer vision.
This means that devices like smartphones, drones, and IoT sensors at the intelligent edge can now process and analyze data in real-time using computer vision and deep learning without relying on cloud computing. By leveraging the power of edge AI, smart devices can perform deep learning and computer vision tasks in real-time. This eliminates the need for data transfer to the cloud, reducing latency and enabling offline operation. Edge AI is particularly beneficial in remote or disconnected environments.

How Edge AI Works
Edge AI, short for Edge Artificial Intelligence, is a powerful technology that brings the capabilities of computer vision and inferencing to edge devices such as cameras. It has various applications in different industries. It works by leveraging machine learning algorithms to process data locally on intelligent edge devices, within an edge computing environment, in real-time, rather than relying on cloud-based servers. This enables intelligent decision-making at the edge using connected devices and cloud AI without the need for constant connectivity. The computer can process data in real-time.
Leveraging Specialized Hardware
To efficiently process data and perform complex computations for real-time applications, Edge AI utilizes specialized hardware such as Graphics Processing Units (GPUs) and Tensor Processing Units (TPUs). This enables inferencing at the end of the edge network. These hardware components are designed to accelerate machine learning tasks in real-time, allowing edge devices to handle sophisticated AI models effectively for applications such as inferencing. Red Hat supports the use of these components.
Training and Deployment of Edge AI Models
Edge AI models are trained on powerful servers using large datasets for real-time applications. These models are designed to deliver efficient and accurate results, making them ideal for a wide range of end-use cases. With the support of Red Hat technology, organizations can leverage the power of Edge AI to enhance their applications and provide real-time insights. During the training phase, these models learn patterns and make predictions based on the provided data in edge computing environments. These predictions are then utilized in various applications at the network edge. Once trained, these models can be deployed directly onto edge devices for real-time inference in applications at the end.
Localized Decision-Making
One of the key advantages of Edge AI is its ability to enable localized decision-making. By processing data locally on edge devices, cloud AI reduces latency and eliminates dependency on continuous internet connectivity for AI applications. This is particularly beneficial in scenarios where real-time decisions need to be made quickly using cloud AI, or when network connectivity may be unreliable or limited for edge computing and AI applications on an edge device.
Benefits of Edge AI Deployment
Edge AI deployment offers several benefits that enhance the performance and efficiency of artificial intelligence systems. Let’s explore these advantages in more detail:
Improved Response Time
By enabling instant decision-making at the edge, Edge AI significantly improves response time. Instead of relying on sending data to the network edge for processing, AI algorithms can run directly on devices or local servers using edge computing. This means that actions can be taken immediately without waiting for data to travel back and forth between the device and the edge computing.
Reduced Bandwidth Usage
One of the key benefits of Edge AI is its ability to reduce bandwidth usage. Only relevant data from edge computing and edge AI devices needs to be transmitted to the cloud, as opposed to sending all raw data for processing. This reduces the amount of network traffic and minimizes latency, making it ideal for applications with limited bandwidth or unreliable network connections in the cloud AI and edge computing domains.
Enhanced Privacy
Edge AI deployment prioritizes privacy by keeping sensitive data localized on the device itself. Since data processing occurs locally in edge computing, there is no need to transmit personal or confidential information to external servers in the cloud AI. This ensures greater control over sensitive data in cloud AI and mitigates potential security risks associated with transmitting information over networks in edge computing.
Increased Reliability
Another advantage of Edge AI deployment is increased reliability. Even in cases where network connectivity is lost or disrupted, edge devices can continue functioning independently. By leveraging edge computing, they can perform computations locally without relying solely on cloud services or internet connectivity. This makes Edge AI particularly valuable in scenarios where uninterrupted operation is critical.
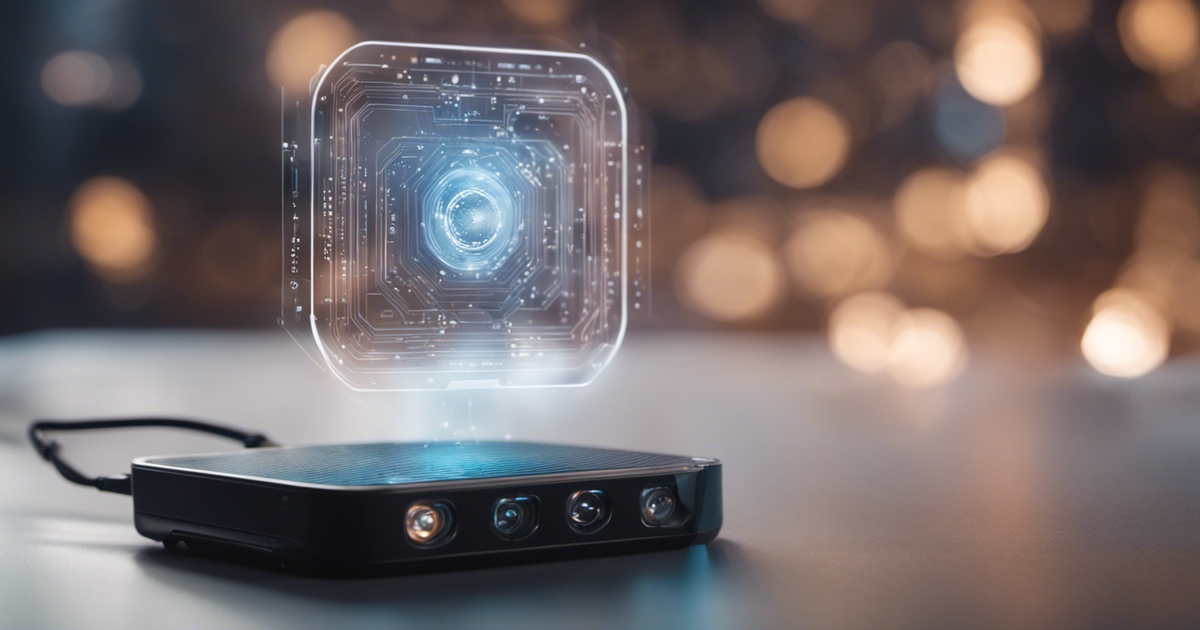
Use Cases of Edge AI in Various Industries
Edge AI, also known as edge artificial intelligence, has found its application in various industries, revolutionizing the way tasks are performed. Let’s explore some of the key use cases of edge AI across different sectors.
Healthcare
In the healthcare industry, edge AI plays a crucial role in enabling real-time monitoring and diagnosis of patients’ vital signs. With the help of AI models and computer vision techniques, medical professionals can analyze data collected from wearable devices or IoT devices at the edge. This allows for the immediate detection of anomalies or changes in patient’s health conditions using edge computing, leading to timely interventions and improved patient outcomes.
Manufacturing
Edge AI is transforming manufacturing processes by facilitating predictive maintenance and quality control on production lines. By deploying AI systems at the edge, manufacturers can monitor equipment performance in real time and detect any potential issues before they lead to costly breakdowns or delays. Edge AI enables quality control by analyzing images captured during production processes, ensuring that products meet desired specifications.
Autonomous Vehicles
In the realm of autonomous vehicles, edge AI plays a critical role in supporting object detection, recognition, and decision-making tasks. By leveraging neural networks and machine learning algorithms at the edge, self-driving cars can quickly identify pedestrians, other vehicles, traffic signs, and obstacles on the road. This enables them to make informed decisions in real time using edge computing and navigate safely through complex environments.
Retail
The retail sector benefits greatly from edge AI as it powers personalized recommendations and inventory management systems. By analyzing customer behavior data collected from various sources such as mobile apps or IoT devices at the edge, retailers can provide tailored product recommendations to individual customers. Moreover, with real-time inventory management powered by edge AI systems, retailers can optimize stock levels based on demand patterns and prevent out-of-stock situations.
Exploring the Relationship between Cloud Computing and Edge Computing
Cloud computing and edge computing are two essential concepts in the world of technology. While edge computing and traditional computing serve different purposes, they work together to optimize performance and scalability in various environments. Edge computing is a decentralized approach that brings computation and data storage closer to the source, reducing latency and bandwidth usage. This allows for faster processing and real-time analytics at the edge of the network. By offloading some tasks to edge devices, traditional computing can focus on more complex processing and analysis, resulting in improved overall system performance and scalability. Let’s take a closer look at how edge computing and these two concepts relate to each other.
Cloud Computing: Centralized Storage and Processing
Cloud computing involves the centralized storage and processing of data in remote servers, often referred to as cloud data centers. These offsite data centers provide a centralized cloud computing facility where organizations can store their data and run applications. The main advantage of cloud computing is its ability to offer vast storage capacity, high computational power, and accessibility from anywhere with an internet connection.
Edge Computing: Complementing Cloud Computing
On the other hand, edge computing complements cloud computing by performing computations closer to where the data is generated or needed. Instead of relying solely on a centralized cloud environment, edge servers are deployed at the network edge or even directly on connected devices. Edge computing allows for faster processing, reduced latency, improved security, and enhanced privacy.
A Hybrid Model for Optimal Performance
In practice, both cloud computing and edge computing work together in a hybrid model that combines their strengths. Certain tasks can be processed locally at the network edge using edge servers or devices with sufficient computational capabilities. Meanwhile, more complex computations or large-scale data analysis can still be offloaded to the centralized cloud infrastructure, but with the rise of edge computing, these tasks can now be performed closer to the source of data.
This hybrid approach ensures optimal performance in edge computing by leveraging both local processing power and the scalability of cloud resources when needed. Edge computing enables real-time decision-making in scenarios where low latency is crucial, while also benefiting from the cost-effectiveness and flexibility offered by cloud services.
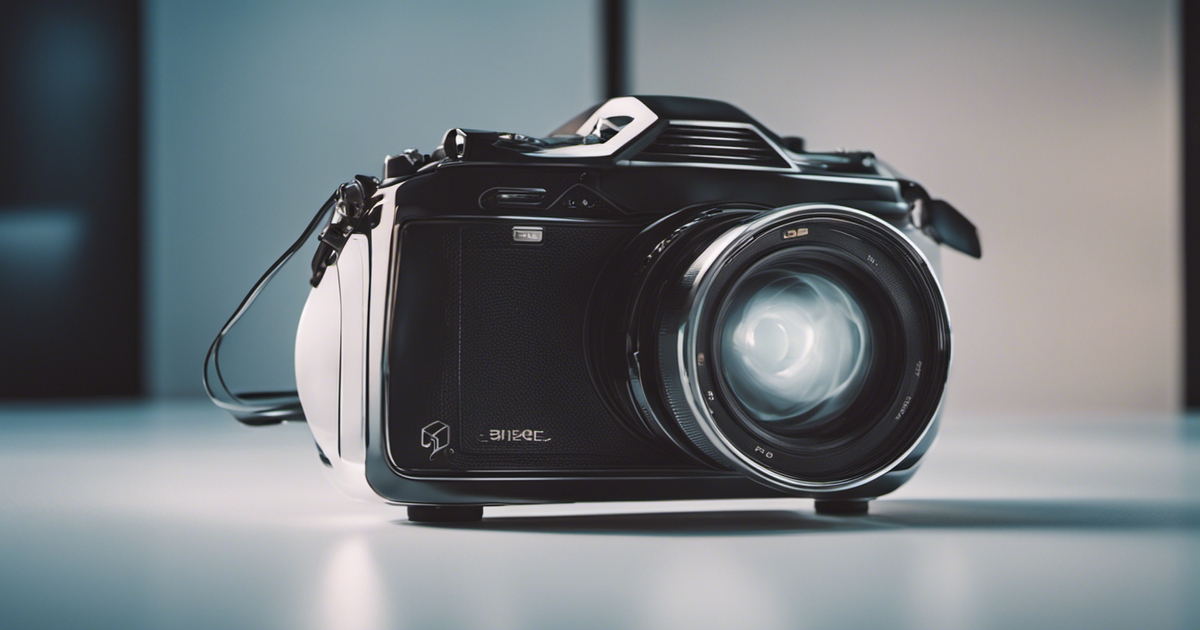
Real-time Data Processing and Efficiency in Edge AI
Real-time data processing is a key feature of edge AI that allows for instant decision-making without relying on external servers. This means that data can be processed and analyzed immediately, providing quick insights and enabling faster response times. With edge AI, the efficiency lies in its ability to process data locally, reducing the need for constant data transfer.
One of the main advantages of edge AI is its ability to eliminate latency associated with cloud computing. By processing data at the edge, closer to where it is generated, there is no need to wait for data to travel back and forth from the cloud. This significantly reduces response times, making it ideal for time-sensitive applications such as security cameras or autonomous vehicles.
Edge AI helps reduce network congestion and bandwidth requirements by processing data locally. Instead of sending all the raw sensor data to a central server or cloud for analysis, edge devices can perform initial processing and only send relevant information. This not only saves bandwidth but also improves overall system performance.
The use of machine learning algorithms and inference engines at the edge enables smart devices to make intelligent decisions without relying on continuous connectivity to the internet. This ensures that even when there are connectivity issues or limited network access, local processes can still function effectively.
Security and Privacy Considerations in Edge AI
Edge AI brings with it several advantages. Let’s explore how this technology addresses these crucial concerns.
Enhanced Security
One of the major benefits of Edge AI is its ability to enhance security. By keeping sensitive data on the device itself, rather than transmitting it over networks, Edge AI minimizes the risk of data breaches. This means that information is processed locally without ever leaving the device, reducing vulnerability to external threats.
Minimized Data Breach Risk
With Edge AI, personal data can be processed on-device without being shared with external servers. This significantly improves privacy by minimizing the potential for unauthorized access or misuse of sensitive information. By leveraging local processing capabilities, Edge AI ensures that data remains secure within the confines of the device.
Improved Privacy
In addition to enhanced security measures, Edge AI also offers improved privacy protection. By processing personal data locally on device, there is no need for it to be transmitted or stored on external servers. This eliminates concerns about third-party access or potential breaches during data transmission. Users can have peace of mind knowing that their personal information remains within their control.
Secure Communication Protocols
To ensure the safe transmission of data between edge devices and cloud services, secure communication protocols are employed in Edge AI systems. These protocols establish encrypted channels for transferring data securely between devices and cloud servers, safeguarding against unauthorized interception or tampering.
The Future of Edge AI Technology
In conclusion, edge AI is revolutionizing the way we process and analyze data. By bringing the power of artificial intelligence to the edge devices themselves, we can achieve real-time data processing, enhanced efficiency, and improved security and privacy. With edge AI, you no longer have to rely solely on cloud computing for complex tasks. Instead, you can harness the capabilities of local devices to perform advanced analytics right at the source of data generation with an edge AI solution. So, what is edge AI?
As technology continues to advance, we can expect even greater integration of edge AI in various industries. From cutting edge AI autonomous vehicles that make split-second decisions on the road to forward edge AI smart cities that optimize resource allocation in real time, the potential applications of Starkey Livio Edge AI are vast. Embracing edge AI opens up a world of possibilities where devices become smarter and more autonomous.
To stay ahead in this rapidly evolving landscape, it’s crucial to keep yourself informed about the latest developments in edge AI. Explore how your industry can benefit from this technology and consider integrating it into your processes. By embracing edge AI today, you’ll be well-positioned for a future where intelligent devices work seamlessly together to drive innovation and efficiency.
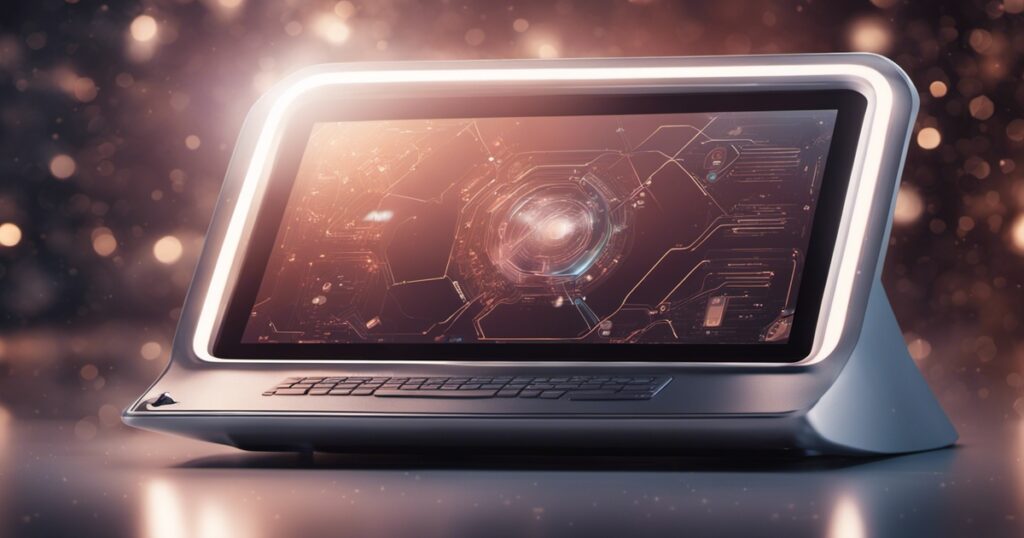
FAQs
What are some examples of industries using Edge AI?
Edge AI has numerous applications across various industries. Some examples include:
- Healthcare: Remote patient monitoring and personalized treatment plans.
- Manufacturing: Predictive maintenance and quality control.
- Retail: Real-time inventory management and personalized shopping experiences.
- Transportation: Autonomous vehicles and traffic optimization.
- Agriculture: Crop monitoring and precision farming techniques.
Is Edge AI more secure than cloud computing?
Edge AI offers enhanced security compared to relying solely on cloud computing. By processing data locally on edge devices instead of sending it over networks to centralized servers, there is less risk of sensitive information being intercepted or compromised during transmission.
Can Edge AI improve energy efficiency?
Yes! Edge AI can significantly improve energy efficiency by reducing reliance on cloud computing. By performing data processing and analytics locally on edge devices, it reduces the need for constant data transfer to remote servers, resulting in reduced energy consumption.
How does Edge AI handle real-time data processing?
Edge AI is specifically designed to handle real-time data processing. By leveraging the computational power of edge devices, it can quickly analyze incoming data and make instant decisions without relying on a centralized cloud infrastructure. This enables faster response times and more efficient operations.
What are the privacy considerations with Edge AI?
Privacy is a crucial aspect of Edge AI deployment. Since data processing happens locally on edge devices, there is less reliance on transmitting sensitive information to external servers. However, it’s essential to implement robust security measures at the device level to protect against potential breaches or unauthorized access.
Can Edge AI work without an internet connection?
Yes! One of the key advantages of Edge AI is its ability to function even in environments with limited or no internet connectivity. By processing data locally on edge devices, it can operate independently without relying on continuous network connectivity.
How does Edge AI complement cloud computing?
Edge AI and cloud computing are complementary technologies that work together to optimize overall system performance. While edge devices handle real-time processing and decision-making, cloud computing provides additional resources for complex tasks that require extensive computational power or historical analysis. The combination of both allows for a seamless integration of local intelligence and centralized capabilities.